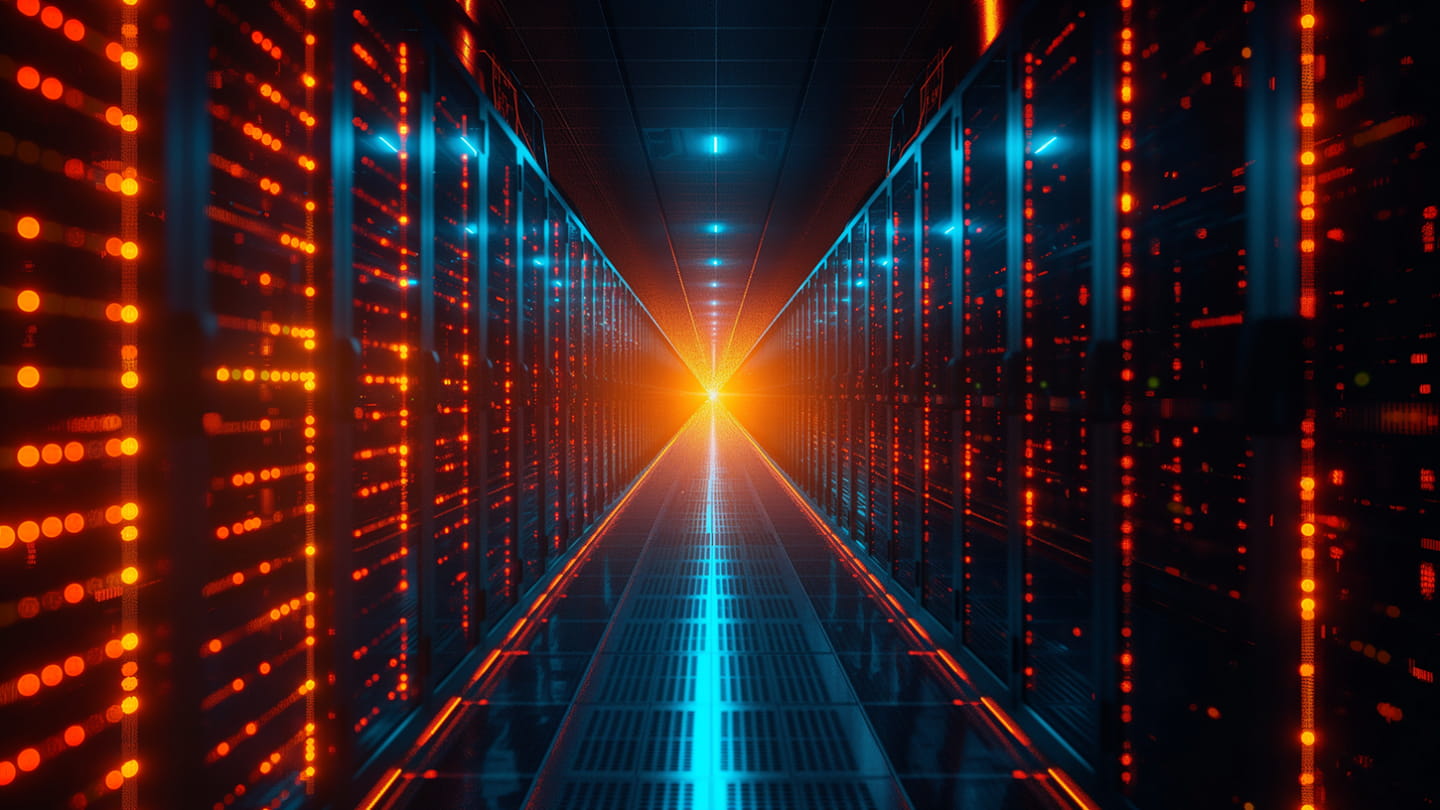
AI’s Hunger for Data: Fueling the Race for Cheaper Cloud Storage
Artificial intelligence ( #AI ) is reshaping industries, but its voracious appetite for data is presenting a challenge: efficiently and affordably storing ever-growing information pools. This insatiable need for more data storage is undeniably propelling the development of increasingly cost-effective and innovative cloud storage solutions.
The AI-Data Connection
Advanced AI algorithms, particularly in domains like natural language processing and computer vision, require training on massive datasets to enhance their accuracy and capabilities. For tasks like facial recognition, generating human-quality text ( i.e. #ChatGPT ), or powering cutting-edge image generators like DALL-E and Stable Diffusion, vast amounts of storage are crucial.
This poses a problem. Traditional cloud storage providers can be expensive when scaling to accommodate AI’s requirements. Consequently, companies and researchers are seeking ways to curb these costs while maintaining storage performance.
Enter the New Wave of Cloud Storage
AI’s demand for data storage is a key catalyst behind trends reshaping the cloud:
#Decentralized Storage: Projects like #Filecoin and #Storj embrace blockchain technology to create distributed storage networks. By tapping into unused storage around the world, they promise greater cost-effectiveness and scalability.
Data Efficiency Optimizations: AI is helping with AI! Novel AI-powered techniques identify unused or redundant data while compressing large files intelligently without compromising quality, lowering storage footprints significantly.
Emerging Storage Technologies: Research into DNA-based data storage and photonic approaches could fundamentally shift the long-term storage landscape, promising massive information density in tiny form factors.
io.net: Disrupting the Cloud with a Decentralized Solution
Traditional cloud options might struggle to meet the unique demands of machine learning. Recognizing this, io.net offers a compelling alternative as a state-of-the-art decentralized computing network. The benefits to machine learning engineers are significant:
Affordability at Scale: Access distributed cloud clusters at drastically reduced costs compared to centralized providers.
Addressing Modern ML Needs: ML applications are inherently well-suited to parallel and distributed computing. io.net’s network optimizes the usage of multiple cores and systems to handle larger datasets and more complex models.
Overcoming the Constraints of Centralized Clouds:
Fast Access: Bypass provisioning delays with rapid access to GPUs, streamlining project launch.
Tailored Solutions: Enjoy customization, choosing precise GPU hardware, locations, and security parameters – something often limited with traditional providers.
Controlled Costs: io.net brings significant cost savings, making large-scale ML projects far more attainable.
The DePIN Difference
io.net unlocks its advantages through an innovative DePIN (Decentralized Physical Infrastructure Network). By pooling underutilized GPUs across data centers, crypto miners, and related projects, io.net builds a scalable network with impressive capacity. ML teams gain on-demand power while contributing to a system based on accessibility, customization, and efficiency.
Key Applications for ML
With io.net, engineers can effortlessly scale across GPUs while the system orchestrates scheduling and fault tolerance. It supports crucial ML-focused tasks:
Batch Inference and Model Serving: Parallelize inference across a distributed GPU network.
Parallel Training: Break free from single-device constraints with batch training and parallelization techniques.
Parallel Hyperparameter Tuning: Streamline and simplify model fine-tuning experiments leveraging checkpointing and advanced parameter search capabilities.
Reinforcement Learning: Tap into an open-source library and production-grade capabilities for reinforcement learning applications.
The Privacy Factor: Considerations Amidst Innovation
The relentless demand for data to power AI also spotlights privacy concerns. Storage choices have implications for the safeguarding of potentially sensitive information. Consider:
Data Governance: Establish clear governance around ownership and access rights, including regulatory compliance across jurisdictions.
Encryption and Anonymization: Robust encryption and anonymization are vital, particularly for sensitive information.
Service Provider Responsibility: Providers play a crucial role in protecting data with strong security measures.
Balancing Performance and Usability
Decentralized and emerging storage solutions face tests related to day-to-day AI workloads. Consider:
Speed and Latency: Training AI models can’t be impeded by sluggish data access.
Reliability: Unplanned downtime could disrupt AI work.
Ease of Integration: Storage solutions need to integrate seamlessly into existing AI development flows.
Is Tech Ready for AI’s Appetite?
The race for innovative data storage continues, undoubtedly influenced by AI’s increasing appetite. Can our technological advancements truly keep up with AI’s ever-growing requirements? Will AI systems effectively interpret and leverage all this accessible data? Where do we go from here?
The relationship between AI and data storage is an ongoing story. Will further innovations create the infrastructure necessary for a future where AI’s immense potential is unhindered by storage limitations, all while preserving data security and privacy? These are questions that the industry must continuously grapple with.